Voices: artificial intelligence, community and climate justice with Joycelyn Longdon
- yang zhao
- Sep 12, 2022
- 2 min read
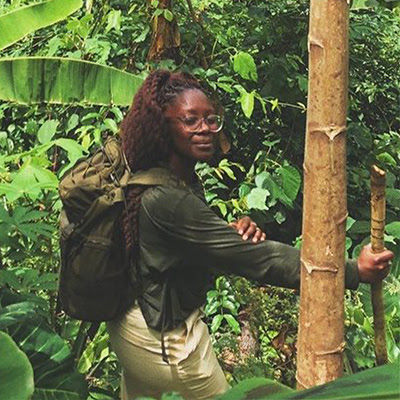
What fascinates you about the intersection of artificial intelligence (AI) and climate change, and why did you choose this topic for your PhD?
AI is used as an umbrella term and can mean anything from email marketing to self-driving cars to robotics to medical diagnosis. Sometimes the phrase itself polarises people, and although my programme is called AI for the study of environmental risks (AI4ER), AI is—as described by Kate Crawford—a phrase that is used choicely to engage and make people excited. Truly what I am doing is machine learning.
I am passionate about the intersection of technology and climate change specifically for forest conservation, but from a perspective of writing a new story of how this should be participatory and grounded in sociology and justice.
I am not as technic-positive as people might expect from a computer scientist, and I don't think that AI is going to save the world. But I am fascinated by the power to use technology, statistics, mathematics and computing to give us better information. Not only information for the sake of information, but information that can tangibly be applied to real-world issues and solve real-world problems and support real people.
format_quote
When a machine learning algorithm chooses who goes to prison or who is searched by the police based on skin colour, it is a bit more obvious where bias can come in. But in the environmental space people are very happy to believe that there is no instance of bias and it is a futile cause.
My PhD title is currently: “Monitoring guardian forests with bioacoustics, machine learning and local ecological knowledge”. This is bringing together the fields of computing, ecology, conservation and sociology to look at how we build community-led technology projects to conserve tropical forests. The way bioacoustics work is you have audio sensors that record the soundscape of a forest and you can use machine learning to identify instances of logging or anthropogenic sound. Tracking [these types of sounds] helps local communities or local authorities have a good idea about the prevalence of human activity in the forest. Or you can use machine learning to identify and monitor certain species and model where they are spatially and temporally to figure out where they are going in the forest, when they are going there, how much they are calling, [whether this] is normal for their species [or] lower, why it is lower, [whether there] are places they are not going, [and] why. My project looks at building a framework for [this type of tool and process] to be community-led and community-involved, ensuring the community has agency over how the project is done and that it’s answering questions that are salient not only to the conservation or policy community but also to the local community.
Comentarios